
Stable DiffusionとUnstable Illusion
Stable Diffusion流行ってますよね。
これについては以下記事等で詳しく説明されていますのでご参照ください。
それで我々はStable Diffusionをオマージュして
Unstable Illusionをつくりました。
Stable Diffusion = 安定的な拡散
↑
に対して
↓
Unstable Illusion = 不安定な幻視
です。
というのも
Stable Diffusionをただ扱うだけなら誰でもやっているので
ひと手間加えてそれっぽいことをしようと試みた次第です。
何をしたかと言うと
Stable Difussionで生成した画像に
TensorFlowのDeepDreamを使って
過解釈を行って、画像に見いだせるパターンの精度を強化しました。
なんだか、夢は幻覚みたいなものなのと、語呂が良いので、
Unstable Illusionとしました。
『Be Here Now』
皆さん『Be Here Now』を読んだことはありますでしょうか?
20センチほどの正方形。茶色い藁半紙に印刷されたインドの神々、そしてラム・ダスからの警句や啓示めいたメッセージ。1970年代初頭に既存の西洋の物質主義へのカウンターとして広まった東洋思想の先鞭をなす、斬新でヒップでグラフィカルなこの一冊が出版されるやいなや世界中の若者たちに受け入れられ、200万部を超えるベストセラーに。新たな知覚の世界を広げるためのヨガやメディテーション、そして精神世界の入り口としても知られています。
と説明が書かれています。
先ほど紹介したDeepDream
は夢とか幻覚とかの話と繋がっていて
スピってる(スピリチュアルっぽい)というので
『Be Here Now』を参照してみました。
スピリチュアル系のわけのわからない本なので
正直、面白くはなかったですが、
"The big ice cream cone in the sky"
(空に浮かぶ大きなアイスクリーム・コーン)
という怪しくて、なんだかシュールな一文があります。
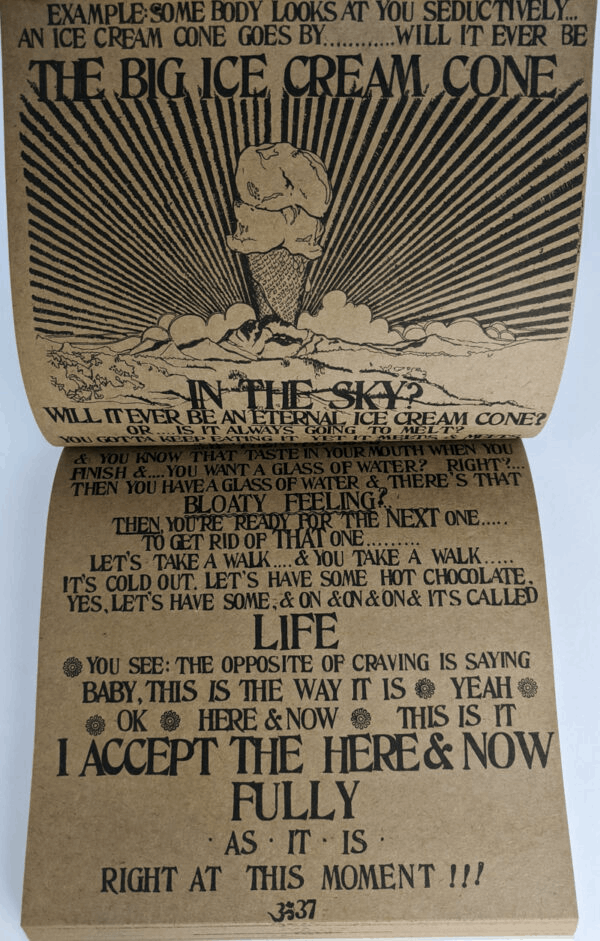
今回はStable DiffusionnとTensorFlow DeepDreamで
この一節の一文The big ice cream cone in the sky
を
抜き出して、画像を生成しようと思います。
準備するもの
- Google Colaboratory GPU環境(※Jupyter NotebookでもOK)
- 配布ソースコード(※一からやりたい方は不要)
使用技術
- Python
- TensorFlow
『Be Here Now』”空に浮かぶ大きなアイスクリーム・コーン”を文字として画像をAI生成する
Stable Diffusion
stable-diffusion-tensorflow
をインストールしましょう。
!pip install git+https://github.com/fchollet/stable-diffusion-tensorflow --upgrade --quiet
!pip install tensorflow tensorflow_addons ftfy --upgrade --quiet
!apt install --allow-change-held-packages libcudnn8=8.1.0.77-1+cuda11.2
生成しましょう。
from stable_diffusion_tf.stable_diffusion import Text2Image
from PIL import Image
generator = Text2Image(
img_height=512,
img_width=512,
jit_compile=False,
)
img = generator.generate(
"The big ice cream cone in the sky",
num_steps=50,
unconditional_guidance_scale=7.5,
temperature=1,
batch_size=1,
)
pil_img = Image.fromarray(img[0])
display(pil_img)
空に浮かぶ大きなアイスクリーム・コーン
が生成されます。
この時点で感心しますね。
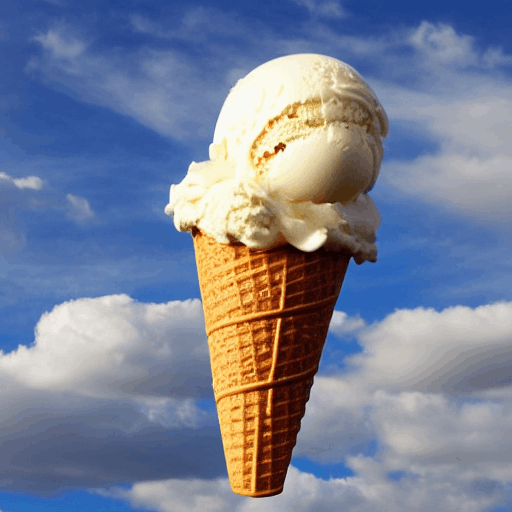
DeepDream
もろもろインポートします。
import tensorflow as tf
import numpy as np
import matplotlib as mpl
import IPython.display as display
import PIL.Image
画像の脱処理をして、表示します。
def download(img, max_dim=None):
return np.array(img)
def deprocess(img):
img = 255*(img + 1.0) / 2.0
return tf.cast(img, tf.uint8)
def show(img):
display.display(PIL.Image.fromarray(np.array(img)))
original_img = download(pil_img, max_dim=500)
show(original_img)
display.display(display.HTML('Image cc-by: <a "href=https://commons.wikimedia.org/wiki/File:Felis_catus-cat_on_snow.jpg">Von.grzanka</a>'))
基盤モデルを作成します。
base_model = tf.keras.applications.InceptionV3(include_top=False, weights='imagenet')
DeepDreamモデルを作成します。
names = ['mixed3', 'mixed5']
layers = [base_model.get_layer(name).output for name in names]
dream_model = tf.keras.Model(inputs=base_model.input, outputs=layers)
損失関数を作成します。
def calc_loss(img, model):
img_batch = tf.expand_dims(img, axis=0)
layer_activations = model(img_batch)
if len(layer_activations) == 1:
layer_activations = [layer_activations]
losses = []
for act in layer_activations:
loss = tf.math.reduce_mean(act)
losses.append(loss)
return tf.reduce_sum(losses)
DeepDreamクラスを作成します。
class DeepDream(tf.Module):
def __init__(self, model):
self.model = model
@tf.function(
input_signature=(
tf.TensorSpec(shape=[None, None, 3], dtype=tf.float32),
tf.TensorSpec(shape=[], dtype=tf.int32),
tf.TensorSpec(shape=[], dtype=tf.float32),
)
)
def __call__(self, img, steps, step_size):
loss = tf.constant(0.0)
for n in tf.range(steps):
with tf.GradientTape() as tape:
tape.watch(img)
loss = calc_loss(img, self.model)
gradients = tape.gradient(loss, img)
gradients /= tf.math.reduce_std(gradients) + 1e-8
img = img + gradients*step_size
img = tf.clip_by_value(img, -1, 1)
return loss, img
クラスを生成します。
deepdream = DeepDream(dream_model)
DeepDream実行関数を作成します。
def run_deep_dream_simple(img, steps=100, step_size=0.01):
img = tf.keras.applications.inception_v3.preprocess_input(img)
img = tf.convert_to_tensor(img)
step_size = tf.convert_to_tensor(step_size)
steps_remaining = steps
step = 0
while steps_remaining:
if steps_remaining > 100:
run_steps = tf.constant(100)
else:
run_steps = tf.constant(steps_remaining)
steps_remaining -= run_steps
step += run_steps
loss, img = deepdream(img, run_steps, tf.constant(step_size))
display.clear_output(wait=True)
show(deprocess(img))
print("Step {}, loss {}".format(step, loss))
result = deprocess(img)
display.clear_output(wait=True)
show(result)
return result
Unstable Illusion
生成しましょう。
dream_img = run_deep_dream_simple(
img=original_img,
steps=100,
step_size=0.01
)
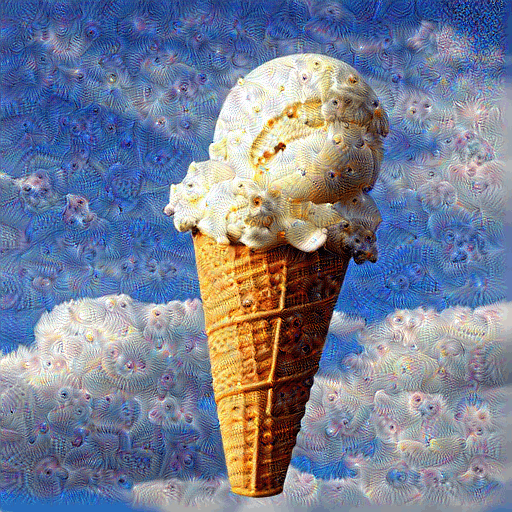
パターンの強度を上げてみましょう。
import time
start = time.time()
OCTAVE_SCALE = 1.30
img = tf.constant(np.array(original_img))
base_shape = tf.shape(img)[:-1]
float_base_shape = tf.cast(base_shape, tf.float32)
for n in range(-2, 3):
new_shape = tf.cast(float_base_shape*(OCTAVE_SCALE**n), tf.int32)
img = tf.image.resize(img, new_shape).numpy()
img = run_deep_dream_simple(img=img, steps=50, step_size=0.01)
display.clear_output(wait=True)
img = tf.image.resize(img, base_shape)
img = tf.image.convert_image_dtype(img/255.0, dtype=tf.uint8)
show(img)
end = time.time()
end-start
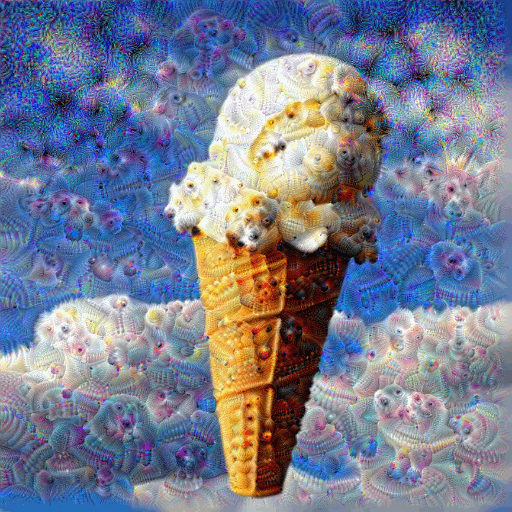
結論
スピってる(スピリチュアルっぽい)…
DeepMagazineはこちら