
Stable Diffusion和Unstable Illusion
Stable Diffusion很流行,不是吗?
请参阅以下文章,了解详细信息。
因此,我们向稳定差异致敬。
我创建了一个可卸载的Illusion。
稳定差异 = 稳定扩散
=
可卸载 Illusion = 不稳定的幻象
它是。
因为
因为每个人都在处理稳定差异,所以
我尝试了一些额外的努力来做它。
我所做的是
使用
TesorFlow 深度分析对
稳定差异生成的图像进行过度解释,以提高图像中可找到的模式的准确性。
不知何故,梦想就像幻觉,因为语言是好的,
我做了一个虚幻的illusion。
『Be Here Now』
你读过《现在在这里》吗?
约20厘米的正方形。 印在棕色的半纸上的印度神,以及拉姆·达斯的警句和启示信息。 这本新颖、时髦、图形化的书,在20世纪70年代初作为对西方现有唯物主义的反面传播,一经出版,就被全世界的年轻人所接受,成为200多万册畅销书。 瑜伽和冥想,以扩大新的感知世界,也被称为精神世界的入口。
并写了描述。
我之前介绍的DeepDream
与梦想或幻觉的故事有关,我
精神错乱(精神上),所以我
参考了“现在在这里”。
说实话,这没什么好笑的,因为
这本书不知道什么是精神系统,
“大冰激
凌的一个”(天空中的大冰淇淋圆锥漂浮在天空中)
可疑,有一个超现实的句子不知何故。
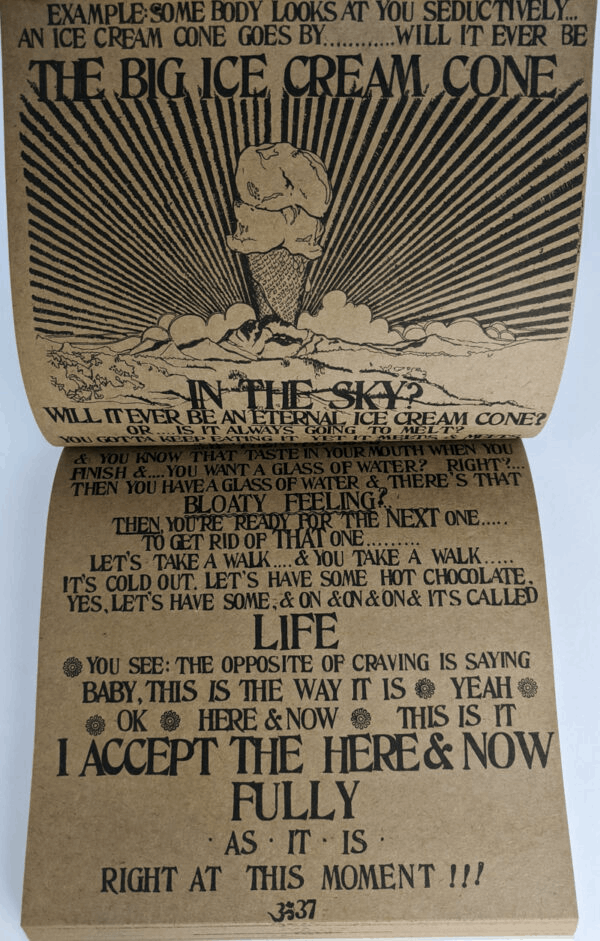
这一次,我将提取一个文本,在稳定差异和登索Flow深度
梦想的这段话
,并生成图像。
要做什么准备
- Google Colaboratory GPU环境(※Jupyter Notebook也OK)
- 分发源代码(*如果您只想从头开始,则不需要)
使用技术
- Python
- TensorFlow
AI 生成以“现在在这里”和“漂浮在天空中的大冰淇淋圆锥体”为字符的图像
Stable Diffusion
安装稳定-差异-tensorflow
。
!pip install git+https://github.com/fchollet/stable-diffusion-tensorflow --upgrade --quiet
!pip install tensorflow tensorflow_addons ftfy --upgrade --quiet
!apt install --allow-change-held-packages libcudnn8=8.1.0.77-1+cuda11.2
让我们生成它。
from stable_diffusion_tf.stable_diffusion import Text2Image
from PIL import Image
generator = Text2Image(
img_height=512,
img_width=512,
jit_compile=False,
)
img = generator.generate(
"The big ice cream cone in the sky",
num_steps=50,
unconditional_guidance_scale=7.5,
temperature=1,
batch_size=1,
)
pil_img = Image.fromarray(img[0])
display(pil_img)
天空中浮现出一个大冰淇淋蛋卷
。
在这一点上,我印象深刻。
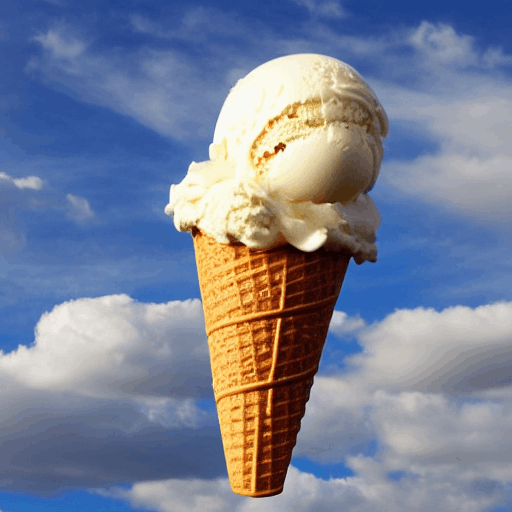
DeepDream
导入所有。
import tensorflow as tf
import numpy as np
import matplotlib as mpl
import IPython.display as display
import PIL. Image
图像被移除并显示。
def download(img, max_dim=None):
return np.array(img)
def deprocess(img):
img = 255*(img + 1.0) / 2.0
return tf.cast(img, tf.uint8)
def show(img):
display.display(PIL. Image.fromarray(np.array(img)))
original_img = download(pil_img, max_dim=500)
show(original_img)
display.display(display.HTML('Image cc-by: <a "href=https://commons.wikimedia.org/wiki/File:Felis_catus-cat_on_snow.jpg">Von.grzanka</a>' ))
创建基础模型。
base_model = tf.keras.applications.InceptionV3(include_top=False, weights='imagenet')
创建深度梦模型。
names = ['mixed3', 'mixed5']
layers = [base_model.get_layer(name).output for name in names]
dream_model = tf.keras.Model(inputs=base_model.input, outputs=layers)
创建损耗函数。
def calc_loss(img, model):
img_batch = tf.expand_dims(img, axis=0)
layer_activations = model(img_batch)
if len(layer_activations) == 1:
layer_activations = [layer_activations]
losses = []
for act in layer_activations:
loss = tf.math.reduce_mean(act)
losses.append(loss)
return tf.reduce_sum(losses)
创建深度梦类。
class DeepDream(tf. Module):
def __init__(self, model):
self.model = model
@tf.function(
input_signature=(
tf. TensorSpec(shape=[None, None, 3], dtype=tf.float32),
tf. TensorSpec(shape=[], dtype=tf.int32),
tf. TensorSpec(shape=[], dtype=tf.float32),
)
)
def __call__(self, img, steps, step_size):
loss = tf.constant(0.0)
for n in tf.range(steps):
with tf. GradientTape() as tape:
tape.watch(img)
loss = calc_loss(img, self.model)
gradients = tape.gradient(loss, img)
gradients /= tf.math.reduce_std(gradients) + 1e-8
img = img + gradients*step_size
img = tf.clip_by_value(img, -1, 1)
return loss, img
生成类。
deepdream = DeepDream(dream_model)
创建深度创建函数。
def run_deep_dream_simple(img, steps=100, step_size=0.01):
img = tf.keras.applications.inception_v3.preprocess_input(img)
img = tf.convert_to_tensor(img)
step_size = tf.convert_to_tensor(step_size)
steps_remaining = steps
step = 0
while steps_remaining:
if steps_remaining > 100:
run_steps = tf.constant(100)
else:
run_steps = tf.constant(steps_remaining)
steps_remaining -= run_steps
step += run_steps
loss, img = deepdream(img, run_steps, tf.constant(step_size))
display.clear_output(wait=True)
show(deprocess(img))
print("Step {}, loss {}".format(step, loss))
result = deprocess(img)
display.clear_output(wait=True)
show(result)
return result
Unstable Illusion
让我们生成它。
dream_img = run_deep_dream_simple(
img=original_img,
steps=100,
step_size=0.01
)
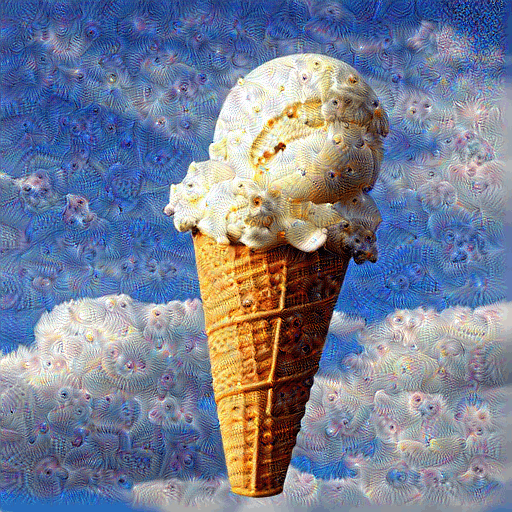
让我们增加图案的强度。
import time
start = time.time()
OCTAVE_SCALE = 1.30
img = tf.constant(np.array(original_img))
base_shape = tf.shape(img)[:-1]
float_base_shape = tf.cast(base_shape, tf.float32)
for n in range(-2, 3):
new_shape = tf.cast(float_base_shape*(OCTAVE_SCALE**n), tf.int32)
img = tf.image.resize(img, new_shape).numpy()
img = run_deep_dream_simple(img=img, steps=50, step_size=0.01)
display.clear_output(wait=True)
img = tf.image.resize(img, base_shape)
img = tf.image.convert_image_dtype(img/255.0, dtype=tf.uint8)
show(img)
end = time.time()
end-start
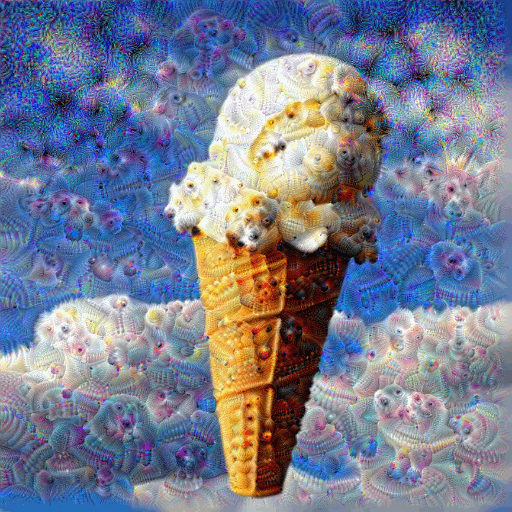
结论
精神(精神)…
DeepMagazine在这里